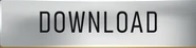

- #Data generator generator#
- #Data generator manual#
- #Data generator software#
- #Data generator series#
Pulling a copy of production data: Don’t ever, ever, ever do this! Just don’t! Not only is there a huge confidentiality risk, there’s also something seriously wrong with the underlying app lifecycle if you’re able to readily pull production data backups into a test environment. If you’re looping through and inserting a million “John Smith” records it’s not going to look right during testing and chances are the black magic within SQL Server’s indexing and query optimisation is not going to behave the way it would with real data. Secondly, it’s not reflective of real data. Have a half dozen tables with multiple columns and relational integrity considerations and there goes a significant amount of time. Hand-crafted scripts: So why not just write some scripts to insert the required number of records? You’ve got two problems with this: Firstly, anything more than a very basic script becomes tedious. You’re inevitably going to either blow huge amounts of time or end up with an insufficient set of data to test against.
#Data generator manual#
Manual creation: Using the app to organically create test data is just plain tedious. Here are some popular approaches which are ultimately all pretty flawed: Thing is though, the way some folks deal with them is rather problematic. The issues above are not new, in fact they’re very well trodden paths. A customer management app, for example, is going to be very hard to test without a good set of customer records. Many of the functions they need to perform in order to fully experience the product are dependent on the presence of data.
#Data generator software#
One of the primary ideas of a test cycle is that it allows the app owner to experience what the software will be like once it rolls out. “It never did that under development or test.” A handful of records in your test environment versus a few million in production will do that.Īnother issue is usability. It’s a common scene for a developer to begin scratching his head when faced with the lethargic performance of an application which has spent some time accruing transactional data. Software has a funny habit of behaving differently once it starts dealing with decent volumes of data. So what’s the problem? Well, there’s a couple of discrete challenges when suitable test data is not available, one of them being performance.
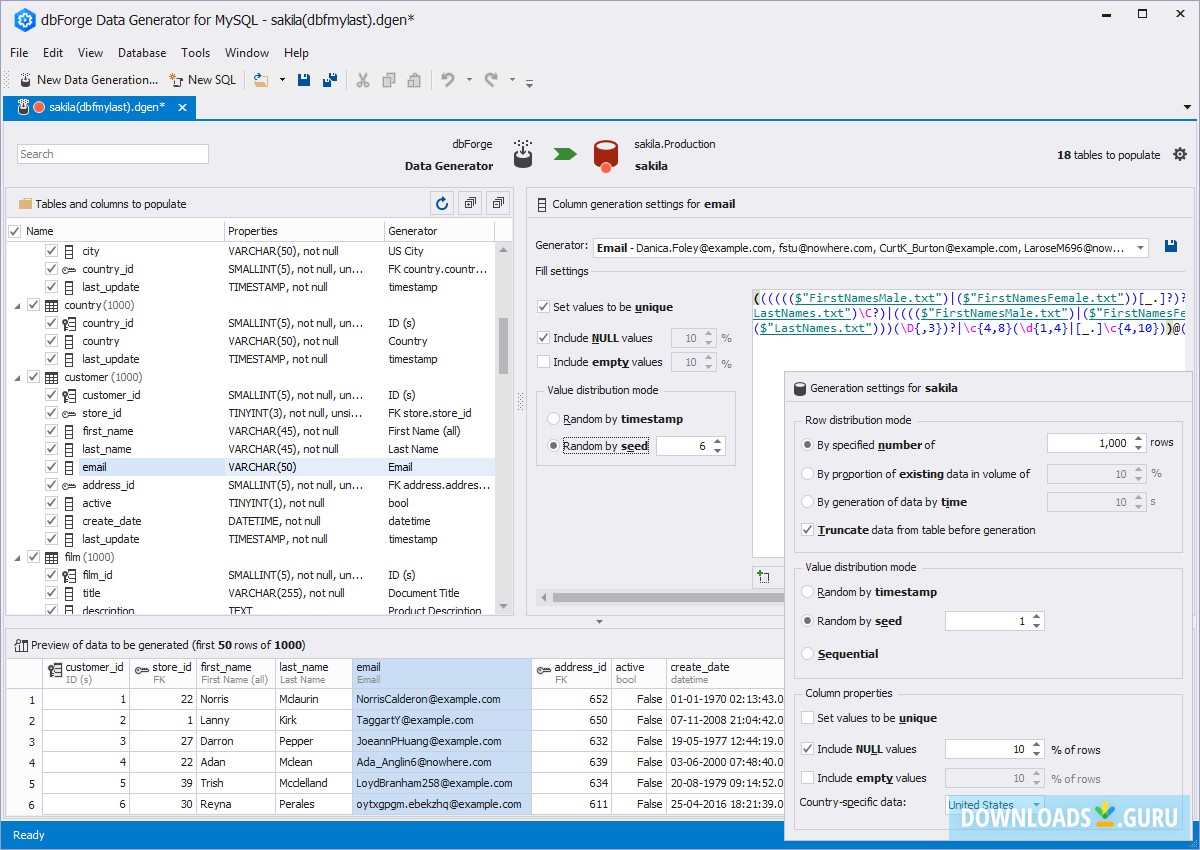
In fact it’s almost magical when you see it in action over your own data schema. And then there’s Red Gate’s SQL Data Generator, which is none of these. There are a whole bunch of counter-techniques for the empty database problem ranging from the tedious to the impractical to the downright ridiculous. The problem is simply this: without data in the test environment which is representative of what you’ll end up with in the production environment, it’s very difficult to properly simulate the way the app will behave after it rolls out.
#Data generator series#
A series of discussions last week got me around to talking about the right way to test a system against a realistic set of data. We show that G-PATE is the first work being able to generate high-dimensional image data with high data utility under limited privacy budgets ($\varepsilon \le 1$). Empirically, we demonstrate the superiority of G-PATE over prior work through extensive experiments. Theoretically, we prove that G-PATE ensures differential privacy for the data generator. In addition, with random projection and gradient discretization, the proposed gradient aggregation mechanism is able to effectively deal with high-dimensional gradient vectors.
#Data generator generator#
In particular, we train a student data generator with an ensemble of teacher discriminators and propose a novel private gradient aggregation mechanism to ensure differential privacy on all information that flows from teacher discriminators to the student generator. Compared to existing approaches, G-PATE significantly improves the use of privacy budgets. Our approach leverages generative adversarial nets to generate data, combined with private aggregation among different discriminators to ensure strong privacy guarantees. In this work, we propose a novel privacy-preserving data Generative model based on the PATE framework (G-PATE), aiming to train a scalable differentially private data generator that preserves high generated data utility. However, large-scale data sharing has raised great privacy concerns. Recent advances in machine learning have largely benefited from the massive accessible training data. Yunhui Long, Boxin Wang, Zhuolin Yang, Bhavya Kailkhura, Aston Zhang, Carl Gunter, Bo Li Abstract Bibtex Paper Reviews And Public Comment » Supplemental
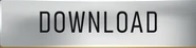